By Meghan McCarthy
As one of the most popular research subjects at this year’s Alzheimer’s Association International Conference (AAIC), machine learning is at the forefront of Alzheimer’s disease and related dementia (ADRD) innovation.
While a seemingly complex topic, machine learning can be thought of as math class for computers. Like students, computers are trained to use different methods, or formulas, to solve a problem.
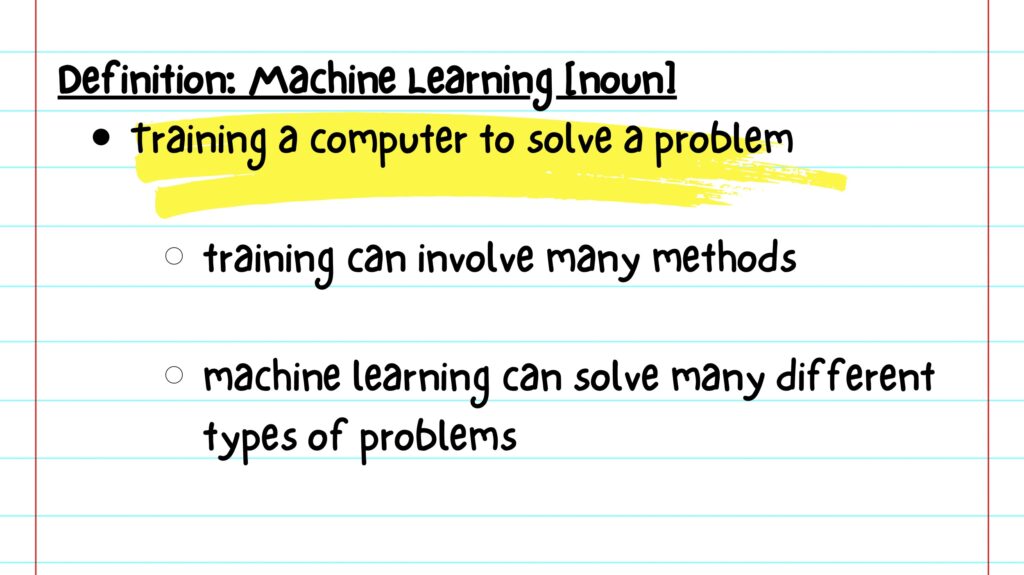
Graphic designed by Meghan McCarthy
There are many types of methods for training, and machine learning is used to solve a wide variety of problems. Most commonly, computers are trained using data sets of patient clinical history, demographic information, and/or brain imaging.
Training in machine learning can be linear.
While there are many types of machine learning, a current and very popular method is known as deep learning. Think of linear training as algebra class and deep learning as (the often dreaded) trigonometry-calculus class.
“In deep learning, a network is built within the machine. This network contains fake neurons, meaning it mimics the networks of cells within your brain,” said Sandy Das, PhD, research assistant professor of neurology at the Perelman School of Medicine and collaborator with PMC Co-Director Dave Wolk, MD. “Ultimately, the network is built to contain thousands or even millions of fake neurons.”
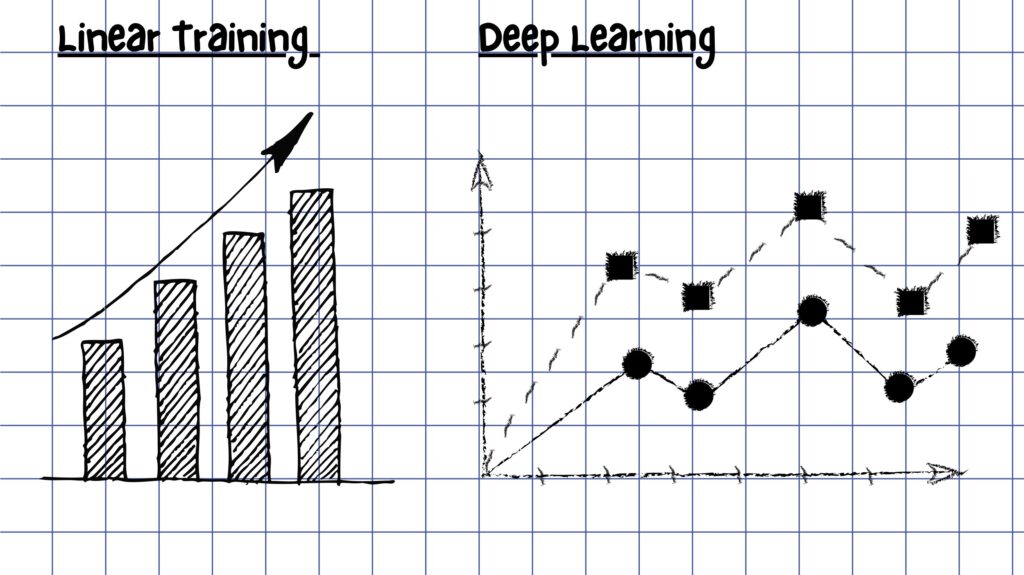
Graphic designed by Meghan McCarthy
These fake neurons connect with one another and can solve more complex problems.
In deep learning, the network has several layers, which allows the machine to capture more complex, non-linear relationships. Ultimately, the computer identifies patterns that humans may not catch.
In ADRD, a common use of machine learning is forecasting a patient’s disease progression. This is known as a type of classification problem.
“For the past 20 years, teams have used machine learning to use imaging and predict the clinical course of a patient,” said Dr. Das.
For example, if a patient is diagnosed with mild cognitive impairment (MCI), will they develop Alzheimer’s disease (AD)? Researchers train computers with neuroimaging such as MRI to predict a patient’s disease progression.
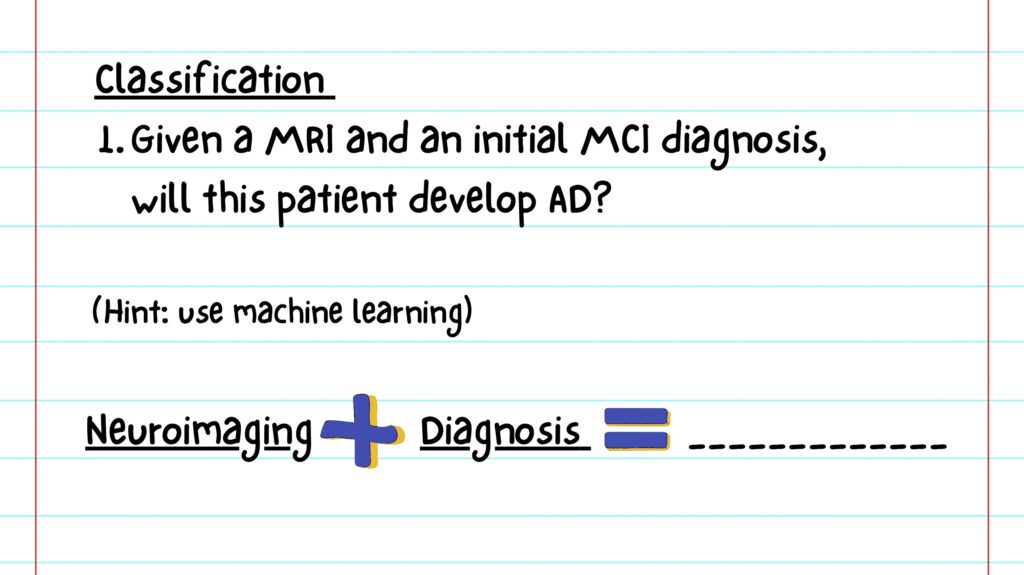
Graphic designed by Meghan McCarthy
They show the computer an MRI of a patient when first diagnosed with MCI and then that patient’s MRI scan 5 to 10 years later.
“By teaching the computer to distinguish between original and current MRIs, the machine learns to predict disease progression,” said Dr. Das.
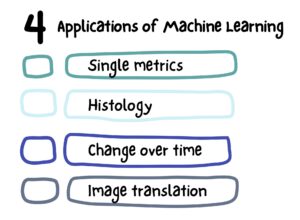
Graphic designed by Meghan McCarthy
Machine learning is used for ADRD problems beyond classification. Examples include determining the biological age of a brain, measuring brain change, and image translation.
“We train a computer to identify nonbiological change, such as different head positions, and discard it when analyzing brain change over time,” said Dr. Das.
This work, in addition to the histology applications, is led by Paul Yushkevich, PhD, a professor of radiology at the Perelman School of Medicine.
The ability to compare brain change over time is especially helpful for clinical trials, which often measure change before and after a drug is given.
In his own lab, Dr. Das collaborates with Dr. Wolk on image translation.
Often, patients get an MRI, but not a PET scan, or vice versa. In image translation, computers are trained to generate one image based off of the other, so patients need fewer scans.
One example of this application generates an amyloid PET scan from an MRI scan.
Ultimately, benefits to this include avoiding the expense, radiation exposure, and time associated with getting another scan.
If a patient does get a PET scan, researchers can compare the synthetic PET to an actual PET to evaluate how well machine learning worked.
Machine learning is not always foolproof. Often, researchers struggle with generalizability, or the general use of their training beyond a specific study.
“Depending on what we are trying to predict, generalizability is not easy,” said Dr. Das. “There’s still a ways to go, but we’ve made incredible progress.”
Dr. Das is a research assistant professor of neurology at the Perelman School of Medicine, and collaborator with Dr. Dave Wolk in Penn’s lab. To learn more about Dr. Das and his work, please click here.