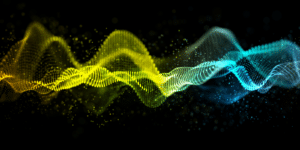
By Meghan McCarthy
Editor’s Note: In light of the 2024 PennAITech Symposium, this article is a part of ongoing coverage on the intersection of technology and dementia.
Depending on where you’re from, phrases and sayings differ. The preference for calling something soda versus pop, hoagie versus sub, bubbler versus water fountain are classic examples that often reflect the state in which one was raised.
Beyond geographical differences, sayings can vary based on racial and ethnic backgrounds.
For instance, Black English, a social dialect with unique expressions and grammar rules, is often used in informal conversations among Black Americans.
When studying speech, these different dialects have an impact on understanding data.
After generating an algorithm that detects cognitive impairment using speech and audio recordings, Maryam Zolnoori, PhD, assistant professor at the Columbia University Medical Center and Columbia University School of Nursing, aims to refine her system through specifically studying speech in Black and African American home healthcare patients.
Last year, the Penn Artificial Intelligence and Technology Collaboratory for Healthy Aging (PennAITech) funded ten pilot studies, including Dr. Zolnoori’s project.
Ultimately, her goal is to change the workflow of cognitive impairment diagnostics in home, primary care, and specialist settings.
Real world experiences prompt pilot study
Dr. Zolnoori’s research during her postdoctoral training at Columbia University centered on using speech to identify risks of adverse outcomes among home healthcare patients.
By recording conversations between patients and nurses, Dr. Zolnoori sought to capture data potentially overlooked by electronic health record systems. She noted that speech patterns often indicated symptoms of cognitive impairment.
“Some patients had difficulty in conversation with nurses,” Dr. Zolnoori said. “In patients with documented memory problems, we noted more pauses in speech, slower speech, and saw that noise in the voice, generated through the vocal cord, sounded different.”
Following these observations, Dr. Zolnoori took action.
To harness the power of speech, her team used audio recordings and to develop a speech processing algorithm, ADscreen, that has a predictive performance score between 85 to 90 percent in detecting cognitive impairment across various audio-recorded datasets.
Specifically, they used audio recordings of patients with and without Alzheimer’s disease from a publicly available dataset at the University of Pittsburg. After analyzing various aspects of the recordings, the team built ADscreen to detect cognitive impairments within patients.
Machine learning algorithms are a type of artificial intelligence that learn from pre-existing data patterns to make predictions for new data.
In Dr. Zolnoori’s case, ADscreen was taught using the publicly available dataset where clinical outcomes of patients were already known. After being taught, the team input new data to see if the algorithm could correctly predict cognitive impairment diagnoses.
ADscreen is designed to enhance the clarity of audio recordings by reducing background noise. It then carefully analyzes both the voice and linguistic characteristics of speech. By applying advanced machine learning techniques, ADscreen generates a detailed assessment that can aid in detecting cognitive impairment. This process transforms complex speech patterns into insightful data, contributing to the early detection of cognitive decline.
Creating an inclusive algorithm
Dr. Zolnoori acknowledged a significant limitation: the dataset primarily included white participants. This raised concerns about the algorithm’s applicability across diverse dialects. To address this gap, Dr. Zolnoori embarked on a pilot study with PennAITech’s support, focusing on Black and African American participants. The study emphasizes the importance of including diverse dialects and speech patterns to refine the algorithm’s effectiveness.
“We proposed this study because we know dialects and pronunciations vary between individuals of different races and ethnicities,” she said. “Cultural phrases and sayings, sentence structure, and grammar all vary between white and Black individuals. To fine tune our algorithm, we really need to have data from Black participants.”
In 2023, Dr. Zolnoori’s team began data collection for the study. The team works with patients that utilize VNS Health NYC-based home health services.
To create a dataset of diverse audio recordings, study research assistants visit Black patients with and without cognitive impairment in their home. Assistants then ask patients two question sets and record the conversations. They also have participants read a passage that includes all phonemes of the English language to better gauge the impact of cognitive impairment on pronunciation.
After the sessions, research assistants have a follow-up call with patients to get information about their health care status.
Changing diagnostic workflow
Currently, Dr. Zolnoori’s funding will cover recruitment of 80 patients. To maximize resources, the team works with patients that have mild cognitive impairments and early dementia but do not include those diagnosed with Alzheimer’s disease.
“We excluded patients with Alzheimer’s disease because we are focusing on developing an algorithm that can detect the early stages of the disease,” Dr. Zolnoori said. “This way, we can provide patients with interventions that are effective in preventing or delaying the progression toward Alzheimer’s.”
In the future, Dr. Zolnoori hopes to include a broader patient demographic, enhancing the algorithm’s precision and applicability.
Ultimately, she hopes ADscreen could boost accessibility of diagnostic resources for patients with Alzheimer’s disease and related dementias (ADRDs).
“We don’t need to wait for a patient to see a neurologist, because usually patients ignore or are not aware of early-stage disease symptoms,” said Dr. Zolnoori. “Access to specialist appointments can be limited. If we can record conversations with nurses and patients even in simple interactions like obtaining blood pressure, their speech can be analyzed.”
In this scenario, if speech indicated qualities of cognitive impairment, Dr. Zolnoori envisions a model where nurses and primary care physicians are notified and asked to complete follow-up questionnaires with patients.
This goal comes with several challenges, including understandable concerns from clinicians about how audio recordings could be misused. For example, some nurses have been hesitant in fear of recordings being shared with managers and then being used to evaluate job performance.
“If we really want to implement this, we need support from managers and policy makers and we need to educate clinicians on the safety and security of this work,” she said.
While there are challenges to overcome, Dr. Zolnoori is driven by personal motivations.
Her aunt first showed symptoms of Alzheimer’s at the age of fifty-nine. A close pair, Dr. Zolnoori picked up on the way her aunt’s voice changed depending on her emotional state and disease progression.
As a loved one, she has lived out the impact of ADRDs. As a researcher, she’s excited to make a difference for families like her own.
“The goal of all of this is to integrate this algorithm into clinical workflow to improve screening and quality of care of patients with cognitive impairment and avoid negative outcomes,” Dr. Zolnoori said.
To read about ADscreen’s development, please click here.
To watch Dr. Zolnoori presention: “AI to Screen for Early-Stage Dementia and Other Risks,” please click here.
To further learn about Dr. Zolnoori’s work, please view a recent Colombia Engineering symposium presentation here.